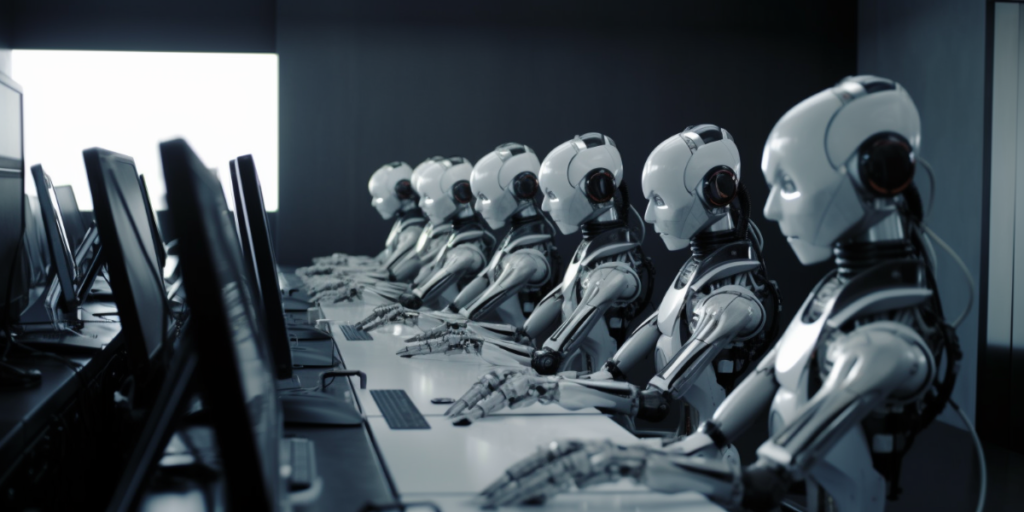
Note: The following post is an elaboration on a recent advisory conversation I help with some institutional investors in the payments space. If you'd like to book me for a consultation or other engagement, check my offerings here.
Here’s the thing, practical, large scale deployments of AI have been used in payments fraud detection since the 1990s. In fact, payments fraud has long been seen as one of the obvious killer apps and ready-adopters for every stage of AI, from neural nets taking over from rules based systems in the 90s. Growth of ecom and attendant fraud, drove payments adoption of machine learning and supervised then unsupervised models through the 00’s, then deep learning and reinforcement learning through the 2020s.
So are large language models and generative AI just more of the same. Well, I think there is reason to argue that this time could be different.
a) Generative AI is not just for the good guys. Watch for a step function acceleration in the volume, effectiveness and new threat vectors for payments fraud. Especially smaller merchants, institutions and processors are going to be increasingly dependent on vendors to keep up in the arms race.
b) Generative AI is not just for the payments business. Businesses of all sizes stand to be benefiting from generative AI providing new value to almost all functions finance, ops, marketing, sales etc. But most mid to small size business will be buying solutions that integrate AI, and AI benefits from as much contextual data it can use and understand about the business. So advantage here to the ongoing super-trend integrated SaaS platforms like Shopify, Square, vertical platforms like Toast and platform-enablers like Stripe. Vs monoline providers like legacy PoS providers or legacy payments acquiring/processing.
Generative AI product opportunities in payment fraud detection
- Pattern Recognition and Anomaly Detection: Large language models can be trained on transaction data to understand normal patterns and recognize anomalous transactions. I’d expect these improvements to be moderately incremental, not disruptive. But LLM ability to understand complex patterns could potentially allow them to identify sophisticated fraud strategies that simpler models might miss.
- Synthetic Data Generation: Generative AI models can be used to create synthetic transaction data that mirrors the properties of real transaction data. This synthetic data can be used to train other machine learning models for fraud detection, particularly in cases where there may be limited examples of certain types of fraud. Actually, being able to test any code in fintech against realistic production data has always been a pain. Either you are potentially putting real sensitive PAI/PII info at risk or you just not testing realistically. Producing better synthetic test data for fintech could be a whole new product line or startup idea in itself.
- Narrative Generation for Alerts: Large language models can generate detailed, understandable narratives describing why a particular transaction was flagged as potentially fraudulent. This could make it easier for human analysts to understand and act upon the alerts generated by the system. Why did your bank flag and just call you to confirm that ‘suspicious’ transaction? Maybe they don’t even know, gen AI could hypothetically help here with more specific and customized messaging both for internal testing/optimization or for improved customer communications.
- Improved Phishing Detection: AI models could be used to analyze the text content of emails, SMS, or other communication channels to detect phishing attempts related to payment fraud. The models could be trained to recognize the subtle linguistic cues that indicate a message is a phishing attempt. Especially relevant when you consider how generative AI is also going to powering more sophisticated phishing in the hands of adversaries. This area is going to be a case of generative AI continuing to fuel an arms race on both sides of fraud. Possibly fraud/security and platform vendors here are really the only true winners in the long run.
- Adaptive Fraud Strategies Detection: Large models seem to be surprisingly good at performing well even when pushed beyond their original training set. As fraud strategies constantly evolve, large language models with continual learning can adapt over time, understanding new tactics used by fraudsters and adjusting their detection mechanisms accordingly. Again, an important consideration when gen AI is also going to be helping the bad actors be more creative, productive and hypertargeted.
- Multi-modal Fraud Detection: Combining text, transaction data, and potentially other types of data (like user behavior data), large language models can aid in creating a more comprehensive view of user activity and detect intricate fraudulent patterns more accurately.
- Contextual Analysis: Generative AI models can help in understanding the contextual information around transactions. For example, they could analyze the text of a customer support chat to understand if a transaction was disputed by the customer, even if the dispute isn’t formally recorded in the transaction database.
More Adjacent Usecases and themes
- Improving Customer Support and Interaction: Large language models can automate and enhance customer interactions, providing immediate, accurate responses to customer inquiries. This can expedite the resolution process for disputes and chargebacks, making the process more efficient for both the consumer and the merchant or bank. But would you buy a generative service just for payment/fraud related interactions? More likely, integrated platforms that combine payments with the rest of a business CRM might be the winners here.
- Automating Evidence Collection: AI can help automate the process of gathering and analyzing data related to a dispute or chargeback. This can provide faster resolution times and more accurate outcomes, reducing the time and cost involved in handling these cases. This one again could be a whole new product or startup idea. Imagine an integration between gong (the SaaS that automatically captures and transcribes all cs/sales conversations) and Visa’s Verifi (a service that helps resolve disputes before or after they become chargebacks). Generative AI could be so good at resolving common disputes of what a sales agent allegedly promised vs what a customer received.
- Predicting Disputes: AI models could potentially predict disputes and chargebacks based on transaction patterns, allowing for proactive measures to prevent or mitigate these cases. Maybe not a unique usecase for generative AI vs traditional ML/AI techniques. However, the increasing ease of access to models and custom training, could make all sorts of AI usecases easier to put in the hands of more users.
- Tailored Resolution Strategies: Based on historical data and ongoing learning, AI could tailor dispute resolution strategies, ensuring that the most effective methods are used for each individual case.
The Threat Environment side of generative AI
- Ever More Sophisticated Phishing Attacks: Large language models could be used to craft highly sophisticated phishing emails, text messages, or other communications that convincingly mimic the style of legitimate communications from banks, employees/bosses, friends or other trusted parties.
- Impersonation: These models could be used to generate realistic chat or voice messages, potentially impersonating bank officials or customer service representatives, leading to social engineering attacks.
- Data Mining: If given access to sensitive data, large language models could potentially be used to mine that data for personally identifiable information (PII), either to develop hyper-targeted attacks or defeat security questions based on personal information
- Bypassing AI-Based Fraud Detection: If fraudsters can gain an understanding of how an AI-based fraud detection system works, they might be able to use large language models to generate transaction patterns that avoid detection.
- Deepfakes: More advanced AI systems could potentially be used to create realistic video or audio ‘deepfakes’. While not a direct risk to the payment process itself, this could facilitate fraud or identity theft that could indirectly impact the payments industry.
- Automated Hacking Attempts: Large language models, given their ability to understand and generate human-like text, could potentially be used to automate certain types of hacking attempts that rely on exploiting human vulnerabilities, such as password guessing or social engineering attacks.
- A whole new generation of ‘script kids’: Generative AI is just very powerful at helping anyone learn to code and some models may be released or leaked without adequate (or any) safeguards around generating malicious applications