I love the idea of turning old gear and devices into cash for a new computer, a flight or other major purchase I might not have had the budget (or excuse!) to pull the trigger on right now. Trade-in programs can be a huge win-win for consumers and retailers -but- unless you have the scale of an Apple or ATT it’s hard to contemplate how you’d set up and manage a consumer trade-in program yourself.
Now, wanna watch me implement a customer trade-in program to any e-commerce store in less than 5 minutes? Well it’s Friday again, and I’m doing fintech demos. Lets go.
Today I’m taking Canadian fintech darling SELLIT9 for a test drive, and their just -launched their “Sellit9 Trade” product. It’s a fully-managed service that lets consumers trade-in used electronics for immediate store credit at the participating merchant. On the merchant side, the integration is surprisingly painless, esp if you are on Shopify where you can enable Sellit9 Trade with one click in the app store. Operationally, the app rides the rails of existing coupon code methods, so there’s no requires no changes to existing payments processors or checkout flows.
Watch the demo as I mess around with setting it up on a mock store, then run through the consumer experience.
As a payments nerd though, I’m always excited to see the emergence of new forms of tender like BNPL and now Trade-ins-as-service. Like BNPL, Trade-ins are an effectively magic-up additional spending power for consumers. But unlike BNPL, more sustainably and without the issues of debt repayment.
High-growth space, excellent product execution, led by a great team of serial founders Josh Guttman & Oswaldo Alvarez these are all the reasons I originally partnered with Sellit as an advisor and seed investor. If you want to give them a test drive yourself, or get an intro to the founders, drop me a PM.
FintechDemoFriday: Adding Sellit9 Trade-ins to my e-commerce site
MCP, ‘Conduit’ companies, AI agents and what happens when all your customers are now bots?
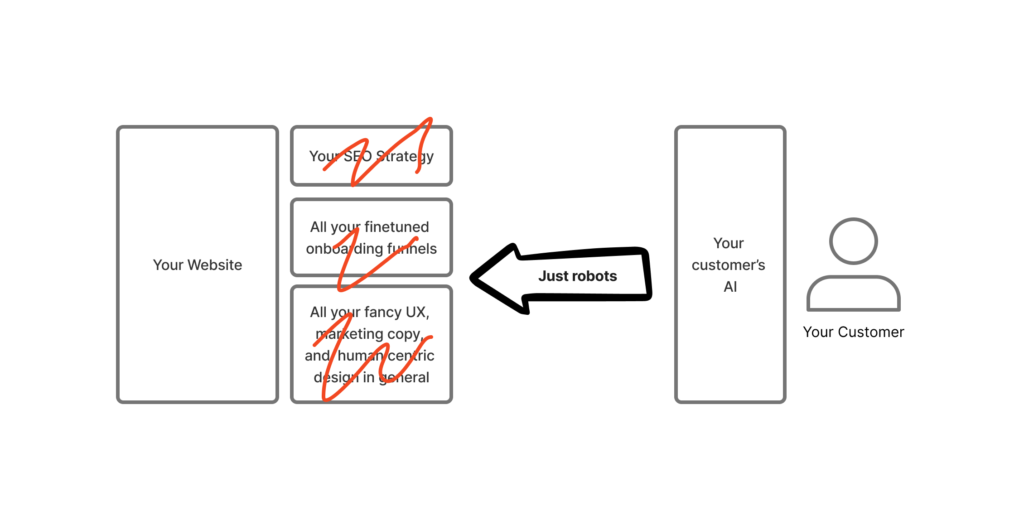
? Substack here by Nick Boesel. AI agents (facilitated by MCP) will quickly become the fastest growing customers interact with services. Disrupting customer-facing channels just like mobile did to web and the web did to MOTO and brick-and-mortar. I don’t think anyone’s ready for this. It’s terra incognita right now for understanding this soon-to-be critical distribution channel. How do you think of customer service design, of landing pages and conversion funnels and GUI design when all your new useragents are literally agents? If you welcome MCP/AI access, or will your customer relationships be usurped by what Nick describes as conduit companies? What will the new AI-led growth playbook look like? I can say for sure, If I were CPO of Expedia or OpenTable or RocketMortgage or Progressive or Geico… I’d be pulling together a hell of SWAT team on this question right now.
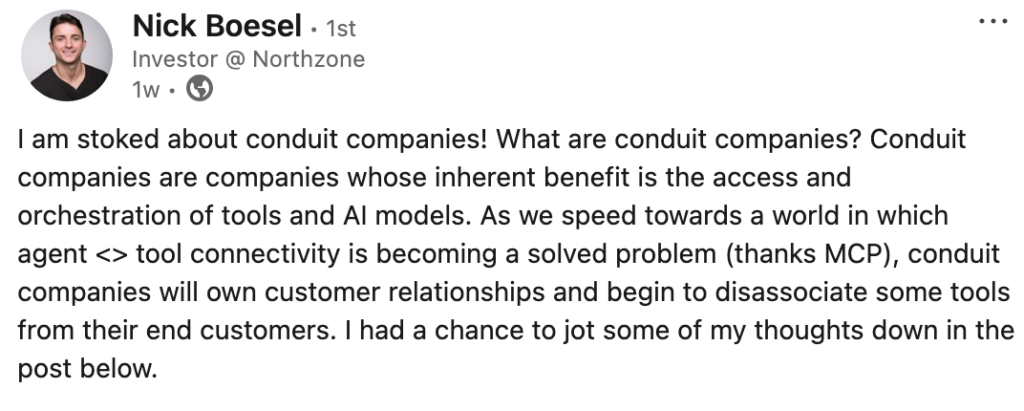
Fintech Demo Friday: Kapti AI Document Ingestion
Got a minute? It’s Fintech Demo Friday! here’s a sneak peek at a new AI toolset for lenders.
In this case were looking at bank statements and docs you might need to validate, digitize and interpret in a lending workflow. My partners over at Blanc Labs build these kinds of solutions all day. But recently they’ve also spun out some of their intelligent document processing, as a standalone product component called Kapti. Here’s me kicking the tires on these kinds of models can do, a little prototype front-end the team put together.
What’s game changing about using LLMs (vs traditional ML/NLP) for these usecases is the potential flexibility to handle data/documents of variable or inconsistent format, but still make sense of them. Further, the potential to better in infer from context how to classify or data enrich individual line items.
What makes an offering like Kapti bank-grade, is the granular observability of the AIs reasoning including confidence levels, customization of business rules as well as the auditability of the entire workflow.
Anyway, enjoy and let me know what you think! Could you use tech like this?